"Estimate performance of SVM for regression"
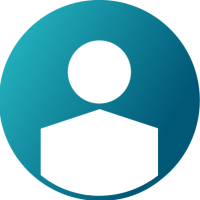
noah977
New Altair Community Member
Hi,
I am building some model files using SVM for REGRESSION (nu-SVR)
I can't seem to find a way to see a performance estimate from this task. Either when training the SVM or using the model file.
I tried the "performance" operator, but it gives me errors.
Any ideas?
I am building some model files using SVM for REGRESSION (nu-SVR)
I can't seem to find a way to see a performance estimate from this task. Either when training the SVM or using the model file.
I tried the "performance" operator, but it gives me errors.
Any ideas?
Tagged:
0
Answers
-
Go thru the following steps:
1) Read your data
2) Learn the model (check the keep the example set)
3) Apply the model
4) Regression performance operator
Here's a modification of the SupportVectorMachine.xml file that you can find in the samples (under Learners)<operator name="Root" class="Process" expanded="yes">
<description text="For many learning tasks, Support Vector Machines are among the best suited learning schemes.They adapt the idea of structural risk minimization and allows for non-linear generalizations with help of kernel functions."/>
<operator name="ExampleSource" class="ExampleSource">
<parameter key="attributes" value="../data/polynomial.aml"/>
</operator>
<operator name="JMySVMLearner" class="JMySVMLearner">
<parameter key="keep_example_set" value="true"/>
<parameter key="kernel_degree" value="3"/>
<parameter key="kernel_type" value="polynomial"/>
</operator>
<operator name="ModelApplier" class="ModelApplier">
</operator>
<operator name="RegressionPerformance" class="RegressionPerformance">
<parameter key="root_mean_squared_error" value="true"/>
</operator>
</operator>0 -
Hi,
please note that the solution earmijo gave calculates only the training error. To get a robust and general performance value you should use a cross validation instead.
Regards,
Tobias0