Due to recent updates, all users are required to create an Altair One account to login to the RapidMiner community. Click the Register button to create your account using the same email that you have previously used to login to the RapidMiner community. This will ensure that any previously created content will be synced to your Altair One account. Once you login, you will be asked to provide a username that identifies you to other Community users. Email us at Community with questions.
Support Vector Machine
Hey guys,
the Random Forest works so far, I'm currently also trying the SVM (Support Vector Machine) as a countercheck. I have filled in a special attribute as Category here, but when I insert the operator Set Role, the SVM operator tells me that a special attribute is missing or insufficient capability. Of course I applied Nominal to Numerical, this requirement must be met. But as I just said, I can't get rid of the error message. As a help the SVM operator offers the "Set Role" operator, but now the svm operator shows me the that the capability is not enough
Thanks for the answers
the Random Forest works so far, I'm currently also trying the SVM (Support Vector Machine) as a countercheck. I have filled in a special attribute as Category here, but when I insert the operator Set Role, the SVM operator tells me that a special attribute is missing or insufficient capability. Of course I applied Nominal to Numerical, this requirement must be met. But as I just said, I can't get rid of the error message. As a help the SVM operator offers the "Set Role" operator, but now the svm operator shows me the that the capability is not enough

Thanks for the answers
BR
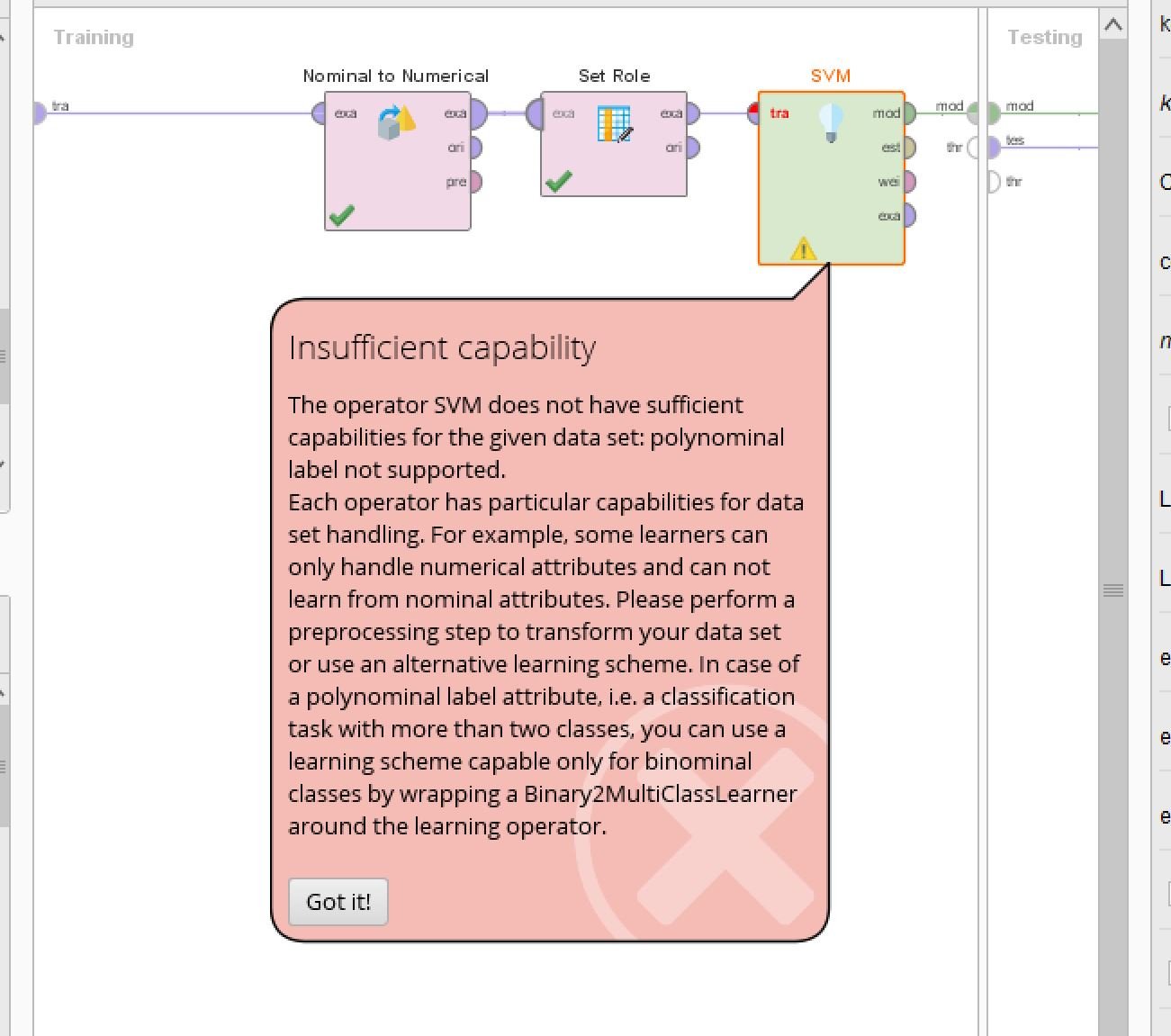
0
Best Answers
-
varunm1 Member Posts: 1,207
Unicorn
If you are trying to predict more than 2 classes then a regular SVM does not work. You can choose Support Vector Machine (LibSVM) for multiclass classification.
Regards,
Varun
https://www.varunmandalapu.com/Be Safe. Follow precautions and Maintain Social Distancing
6 -
IngoRM Employee, RapidMiner Certified Analyst, RapidMiner Certified Expert, Community Manager, RMResearcher, Member, University Professor Posts: 1,751
RM Founder
This is actually desired behavior (so you can still see what the possible values are even if they do not occur in the current value - that is sometimes important). There are a couple of ways to force the re-creation of the meta data for the new data, but my personal favorite is to use a Guess Types operator on that column after the filtering. That will fix the value type to binominal and recreate the meta data.Hope this helps,
Ingo7 -
varunm1 Member Posts: 1,207
Unicorn
Hello @IngoRM
Thanks for informing. That worked. @Flixport, please find the working process with regular SVM in the below process, I added guess types operator and selected Label in operator parameters.<?xml version="1.0" encoding="UTF-8"?><process version="9.2.001">
<context>
<input/>
<output/>
<macros/>
</context>
<operator activated="true" class="process" compatibility="9.2.001" expanded="true" name="Process">
<parameter key="logverbosity" value="init"/>
<parameter key="random_seed" value="2001"/>
<parameter key="send_mail" value="never"/>
<parameter key="notification_email" value=""/>
<parameter key="process_duration_for_mail" value="30"/>
<parameter key="encoding" value="UTF-8"/>
<process expanded="true">
<operator activated="false" class="retrieve" compatibility="9.2.001" expanded="true" height="68" name="Retrieve data_out_select_by_pca_weights" width="90" x="313" y="34">
<parameter key="repository_entry" value="data/data_out_select_by_pca_weights"/>
</operator>
<operator activated="true" class="retrieve" compatibility="9.2.001" expanded="true" height="68" name="Retrieve data_out_pca" width="90" x="45" y="442">
<parameter key="repository_entry" value="../OneDrive_1_4-9-2019/data_out_pca"/>
</operator>
<operator activated="true" class="retrieve" compatibility="9.2.001" expanded="true" height="68" name="Retrieve data_out_select_by_chisq_weights" width="90" x="45" y="187">
<parameter key="repository_entry" value="../OneDrive_1_4-9-2019/data_out_select_by_chisq_weights"/>
</operator>
<operator activated="true" class="concurrency:join" compatibility="9.2.001" expanded="true" height="82" name="Join (2)" width="90" x="179" y="289">
<parameter key="remove_double_attributes" value="true"/>
<parameter key="join_type" value="inner"/>
<parameter key="use_id_attribute_as_key" value="true"/>
<list key="key_attributes"/>
<parameter key="keep_both_join_attributes" value="false"/>
</operator>
<operator activated="true" class="multiply" compatibility="9.2.001" expanded="true" height="82" name="Multiply" width="90" x="313" y="289"/>
<operator activated="true" class="filter_examples" compatibility="9.2.001" expanded="true" height="103" name="Filter Examples" width="90" x="514" y="289">
<parameter key="parameter_expression" value=""/>
<parameter key="condition_class" value="custom_filters"/>
<parameter key="invert_filter" value="false"/>
<list key="filters_list">
<parameter key="filters_entry_key" value="Category.equals.financial"/>
<parameter key="filters_entry_key" value="Category.equals.economic"/>
</list>
<parameter key="filters_logic_and" value="false"/>
<parameter key="filters_check_metadata" value="true"/>
</operator>
<operator activated="true" class="guess_types" compatibility="9.2.001" expanded="true" height="82" name="Guess Types" width="90" x="648" y="289">
<parameter key="attribute_filter_type" value="single"/>
<parameter key="attribute" value="Category"/>
<parameter key="attributes" value=""/>
<parameter key="use_except_expression" value="false"/>
<parameter key="value_type" value="attribute_value"/>
<parameter key="use_value_type_exception" value="false"/>
<parameter key="except_value_type" value="time"/>
<parameter key="block_type" value="attribute_block"/>
<parameter key="use_block_type_exception" value="false"/>
<parameter key="except_block_type" value="value_matrix_row_start"/>
<parameter key="invert_selection" value="false"/>
<parameter key="include_special_attributes" value="true"/>
<parameter key="decimal_point_character" value="."/>
</operator>
<operator activated="true" class="set_role" compatibility="9.2.001" expanded="true" height="82" name="Set Role" width="90" x="782" y="289">
<parameter key="attribute_name" value="Category"/>
<parameter key="target_role" value="label"/>
<list key="set_additional_roles"/>
</operator>
<operator activated="true" class="split_data" compatibility="9.2.001" expanded="true" height="103" name="Split Data" width="90" x="916" y="289">
<enumeration key="partitions">
<parameter key="ratio" value="0.8"/>
<parameter key="ratio" value="0.2"/>
</enumeration>
<parameter key="sampling_type" value="shuffled sampling"/>
<parameter key="use_local_random_seed" value="false"/>
<parameter key="local_random_seed" value="1992"/>
</operator>
<operator activated="true" class="x_validation" compatibility="5.1.002" expanded="true" height="124" name="Validation" width="90" x="1050" y="85">
<parameter key="create_complete_model" value="false"/>
<parameter key="average_performances_only" value="true"/>
<parameter key="leave_one_out" value="false"/>
<parameter key="number_of_validations" value="5"/>
<parameter key="sampling_type" value="2"/>
<parameter key="use_local_random_seed" value="false"/>
<parameter key="local_random_seed" value="1992"/>
<process expanded="true">
<operator activated="true" class="support_vector_machine" compatibility="9.2.001" expanded="true" height="124" name="SVM" width="90" x="313" y="34">
<parameter key="kernel_type" value="dot"/>
<parameter key="kernel_gamma" value="1.0"/>
<parameter key="kernel_sigma1" value="1.0"/>
<parameter key="kernel_sigma2" value="0.0"/>
<parameter key="kernel_sigma3" value="2.0"/>
<parameter key="kernel_shift" value="1.0"/>
<parameter key="kernel_degree" value="2.0"/>
<parameter key="kernel_a" value="1.0"/>
<parameter key="kernel_b" value="0.0"/>
<parameter key="kernel_cache" value="200"/>
<parameter key="C" value="0.0"/>
<parameter key="convergence_epsilon" value="0.001"/>
<parameter key="max_iterations" value="100000"/>
<parameter key="scale" value="true"/>
<parameter key="calculate_weights" value="true"/>
<parameter key="return_optimization_performance" value="true"/>
<parameter key="L_pos" value="1.0"/>
<parameter key="L_neg" value="1.0"/>
<parameter key="epsilon" value="0.0"/>
<parameter key="epsilon_plus" value="0.0"/>
<parameter key="epsilon_minus" value="0.0"/>
<parameter key="balance_cost" value="false"/>
<parameter key="quadratic_loss_pos" value="false"/>
<parameter key="quadratic_loss_neg" value="false"/>
<parameter key="estimate_performance" value="false"/>
</operator>
<connect from_port="training" to_op="SVM" to_port="training set"/>
<connect from_op="SVM" from_port="model" to_port="model"/>
<portSpacing port="source_training" spacing="0"/>
<portSpacing port="sink_model" spacing="0"/>
<portSpacing port="sink_through 1" spacing="0"/>
</process>
<process expanded="true">
<operator activated="true" class="apply_model" compatibility="7.1.001" expanded="true" height="82" name="Apply Model" width="90" x="112" y="34">
<list key="application_parameters"/>
<parameter key="create_view" value="false"/>
</operator>
<operator activated="true" class="performance" compatibility="9.2.001" expanded="true" height="82" name="Performance" width="90" x="246" y="136">
<parameter key="use_example_weights" value="true"/>
</operator>
<connect from_port="model" to_op="Apply Model" to_port="model"/>
<connect from_port="test set" to_op="Apply Model" to_port="unlabelled data"/>
<connect from_op="Apply Model" from_port="labelled data" to_op="Performance" to_port="labelled data"/>
<connect from_op="Performance" from_port="performance" to_port="averagable 1"/>
<portSpacing port="source_model" spacing="0"/>
<portSpacing port="source_test set" spacing="0"/>
<portSpacing port="source_through 1" spacing="0"/>
<portSpacing port="sink_averagable 1" spacing="0"/>
<portSpacing port="sink_averagable 2" spacing="0"/>
</process>
<description align="center" color="transparent" colored="false" width="126">A cross-validation evaluating a decision tree model.</description>
</operator>
<operator activated="true" class="apply_model" compatibility="9.2.001" expanded="true" height="82" name="Apply Model (2)" width="90" x="1184" y="289">
<list key="application_parameters"/>
<parameter key="create_view" value="false"/>
</operator>
<operator activated="true" class="performance_classification" compatibility="9.2.001" expanded="true" height="82" name="Performance (2)" width="90" x="1318" y="391">
<parameter key="main_criterion" value="first"/>
<parameter key="accuracy" value="true"/>
<parameter key="classification_error" value="false"/>
<parameter key="kappa" value="false"/>
<parameter key="weighted_mean_recall" value="false"/>
<parameter key="weighted_mean_precision" value="false"/>
<parameter key="spearman_rho" value="false"/>
<parameter key="kendall_tau" value="false"/>
<parameter key="absolute_error" value="false"/>
<parameter key="relative_error" value="false"/>
<parameter key="relative_error_lenient" value="false"/>
<parameter key="relative_error_strict" value="false"/>
<parameter key="normalized_absolute_error" value="false"/>
<parameter key="root_mean_squared_error" value="false"/>
<parameter key="root_relative_squared_error" value="false"/>
<parameter key="squared_error" value="false"/>
<parameter key="correlation" value="false"/>
<parameter key="squared_correlation" value="false"/>
<parameter key="cross-entropy" value="false"/>
<parameter key="margin" value="false"/>
<parameter key="soft_margin_loss" value="false"/>
<parameter key="logistic_loss" value="false"/>
<parameter key="skip_undefined_labels" value="true"/>
<parameter key="use_example_weights" value="true"/>
<list key="class_weights"/>
</operator>
<connect from_op="Retrieve data_out_pca" from_port="output" to_op="Join (2)" to_port="right"/>
<connect from_op="Retrieve data_out_select_by_chisq_weights" from_port="output" to_op="Join (2)" to_port="left"/>
<connect from_op="Join (2)" from_port="join" to_op="Multiply" to_port="input"/>
<connect from_op="Multiply" from_port="output 1" to_op="Filter Examples" to_port="example set input"/>
<connect from_op="Filter Examples" from_port="example set output" to_op="Guess Types" to_port="example set input"/>
<connect from_op="Guess Types" from_port="example set output" to_op="Set Role" to_port="example set input"/>
<connect from_op="Set Role" from_port="example set output" to_op="Split Data" to_port="example set"/>
<connect from_op="Split Data" from_port="partition 1" to_op="Validation" to_port="training"/>
<connect from_op="Split Data" from_port="partition 2" to_op="Apply Model (2)" to_port="unlabelled data"/>
<connect from_op="Validation" from_port="model" to_op="Apply Model (2)" to_port="model"/>
<connect from_op="Validation" from_port="averagable 1" to_port="result 2"/>
<connect from_op="Apply Model (2)" from_port="labelled data" to_op="Performance (2)" to_port="labelled data"/>
<connect from_op="Performance (2)" from_port="performance" to_port="result 1"/>
<portSpacing port="source_input 1" spacing="0"/>
<portSpacing port="sink_result 1" spacing="0"/>
<portSpacing port="sink_result 2" spacing="0"/>
<portSpacing port="sink_result 3" spacing="0"/>
<description align="left" color="yellow" colored="false" height="139" resized="true" width="565" x="11" y="618">MODEL<br/><br/>Beispiel Anwendung:<br>b. In diesem Beispiel wurde Feld &quot;Topics&quot; als Zielvariable benutzt. Baue ein Model um die restliche Datens&#228;tze zu klassifizieren. Wie sieht die Pr&#228;zision aus?</description>
</process>
</operator>
</process>ThanksRegards,
Varun
https://www.varunmandalapu.com/Be Safe. Follow precautions and Maintain Social Distancing
6
Answers
Is your label binary? If you are trying to predict more than 2 classes then a regular SVM does not work. You can choose Support Vector Machine (LibSVM) for multiclass classification. You can see the number of classes in of your dataset by double-clicking the dataset and going to statistics.
Varun
https://www.varunmandalapu.com/
Be Safe. Follow precautions and Maintain Social Distancing
Can you post the process and dataset? If you cannot give the dataset, you can send in a message?
Varun
https://www.varunmandalapu.com/
Be Safe. Follow precautions and Maintain Social Distancing
I am working on this process of @Flixport. In the process there are 4 classes of data(multi-class), we are filtering for samples related to two classes only but it's still giving the other two classes with zero samples. Not sure if this is some bug.
Process XML below:
<?xml version="1.0" encoding="UTF-8"?><process version="9.2.001">
<context>
<input/>
<output/>
<macros/>
</context>
<operator activated="true" class="process" compatibility="9.2.001" expanded="true" name="Process">
<parameter key="logverbosity" value="init"/>
<parameter key="random_seed" value="2001"/>
<parameter key="send_mail" value="never"/>
<parameter key="notification_email" value=""/>
<parameter key="process_duration_for_mail" value="30"/>
<parameter key="encoding" value="UTF-8"/>
<process expanded="true">
<operator activated="false" class="retrieve" compatibility="9.2.001" expanded="true" height="68" name="Retrieve data_out_select_by_pca_weights" width="90" x="313" y="34">
<parameter key="repository_entry" value="data/data_out_select_by_pca_weights"/>
</operator>
<operator activated="true" class="retrieve" compatibility="9.2.001" expanded="true" height="68" name="Retrieve data_out_select_by_chisq_weights" width="90" x="45" y="391">
<parameter key="repository_entry" value="../OneDrive_1_4-9-2019/data_out_select_by_chisq_weights"/>
</operator>
<operator activated="true" class="retrieve" compatibility="9.2.001" expanded="true" height="68" name="Retrieve data_out_pca" width="90" x="45" y="136">
<parameter key="repository_entry" value="../OneDrive_1_4-9-2019/data_out_pca"/>
</operator>
<operator activated="true" class="concurrency:join" compatibility="9.2.001" expanded="true" height="82" name="Join (2)" width="90" x="179" y="289">
<parameter key="remove_double_attributes" value="true"/>
<parameter key="join_type" value="inner"/>
<parameter key="use_id_attribute_as_key" value="true"/>
<list key="key_attributes"/>
<parameter key="keep_both_join_attributes" value="false"/>
</operator>
<operator activated="true" class="multiply" compatibility="9.2.001" expanded="true" height="82" name="Multiply" width="90" x="313" y="289"/>
<operator activated="true" class="filter_examples" compatibility="9.2.001" expanded="true" height="103" name="Filter Examples" width="90" x="514" y="289">
<parameter key="parameter_expression" value=""/>
<parameter key="condition_class" value="custom_filters"/>
<parameter key="invert_filter" value="false"/>
<list key="filters_list">
<parameter key="filters_entry_key" value="Category.equals.financial"/>
<parameter key="filters_entry_key" value="Category.equals.economic"/>
</list>
<parameter key="filters_logic_and" value="false"/>
<parameter key="filters_check_metadata" value="true"/>
</operator>
<operator activated="true" class="set_role" compatibility="9.2.001" expanded="true" height="82" name="Set Role" width="90" x="715" y="289">
<parameter key="attribute_name" value="Category"/>
<parameter key="target_role" value="label"/>
<list key="set_additional_roles"/>
</operator>
<operator activated="true" class="split_data" compatibility="9.2.001" expanded="true" height="103" name="Split Data" width="90" x="849" y="289">
<enumeration key="partitions">
<parameter key="ratio" value="0.8"/>
<parameter key="ratio" value="0.2"/>
</enumeration>
<parameter key="sampling_type" value="shuffled sampling"/>
<parameter key="use_local_random_seed" value="false"/>
<parameter key="local_random_seed" value="1992"/>
</operator>
<operator activated="true" class="x_validation" compatibility="5.1.002" expanded="true" height="124" name="Validation" width="90" x="1050" y="85">
<parameter key="create_complete_model" value="false"/>
<parameter key="average_performances_only" value="true"/>
<parameter key="leave_one_out" value="false"/>
<parameter key="number_of_validations" value="5"/>
<parameter key="sampling_type" value="2"/>
<parameter key="use_local_random_seed" value="false"/>
<parameter key="local_random_seed" value="1992"/>
<process expanded="true">
<operator activated="true" class="support_vector_machine" compatibility="9.2.001" expanded="true" height="124" name="SVM" width="90" x="313" y="34">
<parameter key="kernel_type" value="dot"/>
<parameter key="kernel_gamma" value="1.0"/>
<parameter key="kernel_sigma1" value="1.0"/>
<parameter key="kernel_sigma2" value="0.0"/>
<parameter key="kernel_sigma3" value="2.0"/>
<parameter key="kernel_shift" value="1.0"/>
<parameter key="kernel_degree" value="2.0"/>
<parameter key="kernel_a" value="1.0"/>
<parameter key="kernel_b" value="0.0"/>
<parameter key="kernel_cache" value="200"/>
<parameter key="C" value="0.0"/>
<parameter key="convergence_epsilon" value="0.001"/>
<parameter key="max_iterations" value="100000"/>
<parameter key="scale" value="true"/>
<parameter key="calculate_weights" value="true"/>
<parameter key="return_optimization_performance" value="true"/>
<parameter key="L_pos" value="1.0"/>
<parameter key="L_neg" value="1.0"/>
<parameter key="epsilon" value="0.0"/>
<parameter key="epsilon_plus" value="0.0"/>
<parameter key="epsilon_minus" value="0.0"/>
<parameter key="balance_cost" value="false"/>
<parameter key="quadratic_loss_pos" value="false"/>
<parameter key="quadratic_loss_neg" value="false"/>
<parameter key="estimate_performance" value="false"/>
</operator>
<connect from_port="training" to_op="SVM" to_port="training set"/>
<connect from_op="SVM" from_port="model" to_port="model"/>
<portSpacing port="source_training" spacing="0"/>
<portSpacing port="sink_model" spacing="0"/>
<portSpacing port="sink_through 1" spacing="0"/>
</process>
<process expanded="true">
<operator activated="true" class="apply_model" compatibility="7.1.001" expanded="true" height="82" name="Apply Model" width="90" x="112" y="34">
<list key="application_parameters"/>
<parameter key="create_view" value="false"/>
</operator>
<operator activated="true" class="performance" compatibility="9.2.001" expanded="true" height="82" name="Performance" width="90" x="246" y="136">
<parameter key="use_example_weights" value="true"/>
</operator>
<connect from_port="model" to_op="Apply Model" to_port="model"/>
<connect from_port="test set" to_op="Apply Model" to_port="unlabelled data"/>
<connect from_op="Apply Model" from_port="labelled data" to_op="Performance" to_port="labelled data"/>
<connect from_op="Performance" from_port="performance" to_port="averagable 1"/>
<portSpacing port="source_model" spacing="0"/>
<portSpacing port="source_test set" spacing="0"/>
<portSpacing port="source_through 1" spacing="0"/>
<portSpacing port="sink_averagable 1" spacing="0"/>
<portSpacing port="sink_averagable 2" spacing="0"/>
</process>
<description align="center" color="transparent" colored="false" width="126">A cross-validation evaluating a decision tree model.</description>
</operator>
<operator activated="true" class="apply_model" compatibility="9.2.001" expanded="true" height="82" name="Apply Model (2)" width="90" x="1184" y="289">
<list key="application_parameters"/>
<parameter key="create_view" value="false"/>
</operator>
<operator activated="true" class="performance_classification" compatibility="9.2.001" expanded="true" height="82" name="Performance (2)" width="90" x="1318" y="391">
<parameter key="main_criterion" value="first"/>
<parameter key="accuracy" value="true"/>
<parameter key="classification_error" value="false"/>
<parameter key="kappa" value="false"/>
<parameter key="weighted_mean_recall" value="false"/>
<parameter key="weighted_mean_precision" value="false"/>
<parameter key="spearman_rho" value="false"/>
<parameter key="kendall_tau" value="false"/>
<parameter key="absolute_error" value="false"/>
<parameter key="relative_error" value="false"/>
<parameter key="relative_error_lenient" value="false"/>
<parameter key="relative_error_strict" value="false"/>
<parameter key="normalized_absolute_error" value="false"/>
<parameter key="root_mean_squared_error" value="false"/>
<parameter key="root_relative_squared_error" value="false"/>
<parameter key="squared_error" value="false"/>
<parameter key="correlation" value="false"/>
<parameter key="squared_correlation" value="false"/>
<parameter key="cross-entropy" value="false"/>
<parameter key="margin" value="false"/>
<parameter key="soft_margin_loss" value="false"/>
<parameter key="logistic_loss" value="false"/>
<parameter key="skip_undefined_labels" value="true"/>
<parameter key="use_example_weights" value="true"/>
<list key="class_weights"/>
</operator>
<connect from_op="Retrieve data_out_select_by_chisq_weights" from_port="output" to_op="Join (2)" to_port="right"/>
<connect from_op="Retrieve data_out_pca" from_port="output" to_op="Join (2)" to_port="left"/>
<connect from_op="Join (2)" from_port="join" to_op="Multiply" to_port="input"/>
<connect from_op="Multiply" from_port="output 1" to_op="Filter Examples" to_port="example set input"/>
<connect from_op="Filter Examples" from_port="example set output" to_op="Set Role" to_port="example set input"/>
<connect from_op="Set Role" from_port="example set output" to_op="Split Data" to_port="example set"/>
<connect from_op="Split Data" from_port="partition 1" to_op="Validation" to_port="training"/>
<connect from_op="Split Data" from_port="partition 2" to_op="Apply Model (2)" to_port="unlabelled data"/>
<connect from_op="Validation" from_port="model" to_op="Apply Model (2)" to_port="model"/>
<connect from_op="Apply Model (2)" from_port="labelled data" to_op="Performance (2)" to_port="labelled data"/>
<connect from_op="Performance (2)" from_port="performance" to_port="result 1"/>
<portSpacing port="source_input 1" spacing="0"/>
<portSpacing port="sink_result 1" spacing="0"/>
<portSpacing port="sink_result 2" spacing="0"/>
<description align="left" color="yellow" colored="false" height="139" resized="true" width="565" x="11" y="618">MODEL<br/><br/>Beispiel Anwendung:<br>b. In diesem Beispiel wurde Feld &quot;Topics&quot; als Zielvariable benutzt. Baue ein Model um die restliche Datens&#228;tze zu klassifizieren. Wie sieht die Pr&#228;zision aus?</description>
</process>
</operator>
</process>
After filtering with Economic and Financial samples, it is still taking agriculture and political but with zero samples. This is the reason regular SVM is throwing an error regarding multiple classes.
Metadata can be found in below link: You can just copy this folder to RM repository.
https://studhsruhrwestde-my.sharepoint.com/personal/mehmet-halit_araz_stud_hs-ruhrwest_de/Documents/Forms/All.aspx?cid=ffa72853-b146-432c-bba3-9dba53bd4ea8&RootFolder=%2Fpersonal%2Fmehmet-halit_araz_stud_hs-ruhrwest_de%2FDocuments%2Fdata&FolderCTID=0x0120006ECEE219C9F2884289BEA6FF46DFD3B3
Thanks
Varun
https://www.varunmandalapu.com/
Be Safe. Follow precautions and Maintain Social Distancing
Varun
https://www.varunmandalapu.com/
Be Safe. Follow precautions and Maintain Social Distancing