Best way to handle imbalanced data
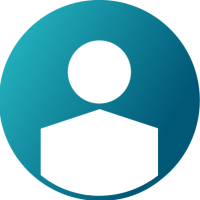
GeezerDoc
New Altair Community Member
I would very much appreciate some guidance. Because RMS has an unusual validation method plus data sampling the test set is small even with hundreds of cases when the class of interest is not balanced. I have read all of the posts about balancing data in the Community Forum and looked at 3 videos on the subject. The current data set I am interested in has 91 patients with dementia and 242 controls. When I uploaded the dataset to WEKA and added SMOTE the AUC increased from .725 to .898, a substantial improvement. I used WEKA simply because SMOTE was an easy filter to add. Several RM forum postings suggest that upsampling techniques will not affect the performance which was not my experience.
I'm stuck with smaller medical datasets to demonstrate/teach binary classification to clinical students. This results in very small numbers in the confusion matrix. What are your recommendations: sample, bootstrapping, SMOTE, etc? Truthfully, I have spent most of my time with TurboPrep and AutoModel so I was unable to figure out how to add SMOTE to the process pipeline. I would appreciate your thoughts.
I'm stuck with smaller medical datasets to demonstrate/teach binary classification to clinical students. This results in very small numbers in the confusion matrix. What are your recommendations: sample, bootstrapping, SMOTE, etc? Truthfully, I have spent most of my time with TurboPrep and AutoModel so I was unable to figure out how to add SMOTE to the process pipeline. I would appreciate your thoughts.
Tagged:
0
Best Answers
-
You need to be careful with SMOTE, especially when you have dramatically unbalanced data or a polynomial label with a daisy chain of SMOTE operators. In this way you may end up with a huge proportion of synthetic data as compared to real data, and hence a biased model, especially that you have a very small data set. Also, ensure that you use SMOTE (and other resampling methods) for model training only and the untouched data for validation, this way your validation partition will reflect the population - alternatively you may need to hand-recalculate all your performance measures as the resampled validation partition no longer agrees with your priors.
0 -
Hello @GeezerDoc
Two things from my side.
1. Models validated on a sampled datasets, some times fail miserably in real-world problems as the imbalance nature cannot be eliminated from real-world settings. Using sampling on the training side can mitigate this to some extent.
2. Kappa value is a good metric to understand whole model performance (balanced or imbalanced datasets).2
Answers
-
I personally would also opt for some smote based analysis, even though you need to be a bit careful to not trick your validation.
@yyhuang recently did quite a lot with it, maybe she can jump in with some best practices?
0 -
You need to be careful with SMOTE, especially when you have dramatically unbalanced data or a polynomial label with a daisy chain of SMOTE operators. In this way you may end up with a huge proportion of synthetic data as compared to real data, and hence a biased model, especially that you have a very small data set. Also, ensure that you use SMOTE (and other resampling methods) for model training only and the untouched data for validation, this way your validation partition will reflect the population - alternatively you may need to hand-recalculate all your performance measures as the resampled validation partition no longer agrees with your priors.
0 -
Thanks for that insight. It seems to me for biomedical datasets imbalanced data remains a huge challenge. There does not seem to be a magic bullet or an absolute consensus on the right approach. For teaching machine learning basics we can warn students that accuracy is misleading and the precision-recall curves may be better than AUCs. What else should we be telling those new to machine learning?1
-
Hello @GeezerDoc
Two things from my side.
1. Models validated on a sampled datasets, some times fail miserably in real-world problems as the imbalance nature cannot be eliminated from real-world settings. Using sampling on the training side can mitigate this to some extent.
2. Kappa value is a good metric to understand whole model performance (balanced or imbalanced datasets).2 -
I am facing the same problem where I have imbalanced dataset (70:30), and I am building models using AutoModel to build my models. How can I handle the imbalanced data, and which step should the 'imbalance data' treatment come into place - after AutoModel or before? Thank you so much. I am totally new to RapidMiner.5