Options
How to add weights to k-NN [SOLVED]
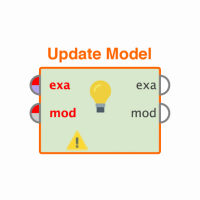

in Help
Hello,
I just sitting in a Rapid-I course I had the question how to add weights to the attributes for an k-NN operator. Now one could answer this satisfying.
So how to weight attributes (e.g.numeric ones) for a weighted distance for the k-NN operator?
I just sitting in a Rapid-I course I had the question how to add weights to the attributes for an k-NN operator. Now one could answer this satisfying.
So how to weight attributes (e.g.numeric ones) for a weighted distance for the k-NN operator?
0
Answers
just let me know when your next break starts and I can explain it to you personally
See you later!
Marius
But what can I do with nominal attributes?
Best regards,
Marius
Nevertheless, what is missing (in my opinion) is a weight input for at least k-NN and Bayes-Operators (since weighting is e.g. a part of the Weka WAODE method, i.e. makes sense there and possibliy also for other operators) to apply attribute weighting in a "natural" way.
Dortmund University?