The RapidMiner community is on read-only mode until further notice. Technical support via cases will continue to work as is. For any urgent licensing related requests from Students/Faculty members, please use the Altair academic forum here.
Your help to enhance my RNN-LSTM solution
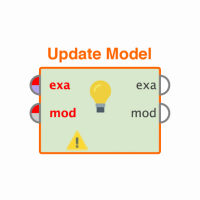

in Help
Hello everyone,
In the first place, I would like to thank you for this helpful RapidMiner community platform that helped me a lot in solving many issues in developing this early prediction model. Thus, I am seeking the RapidMiner community's advice regarding my process.
Actually, I am trying to develop an early prediction model for predicting the value of column D using A, B, and C features. As my data is sequential, I tried two different preprocessing procedures to preprocess the dataset and train the deep learning model for early prediction.
In the first process, I used sequence and batch procedures. I tried to loop through the sequences and create 30% of the sequences for each batch. Then I replaced the final score (the final value) of each batch in the D column at the end of the 30% (for example, if I had 60 sequences in one batch, I sliced out the first 20 sequences and placed the final value of column D (in row 60) at row 20 in column D). After the data preprocessing, I used cross-validation to train the deep learning model.
On the other hand, in the second process, I used the window operator. I looped through the values of the dataset and created a 30% time step window for each batch. Then I placed the final value of each batch as a label for the 30% window. Then I used cross-validation to train the deep learning model.
Actually, I am trying to develop an early prediction model for predicting the value of column D using A, B, and C features. As my data is sequential, I tried two different preprocessing procedures to preprocess the dataset and train the deep learning model for early prediction.
In the first process, I used sequence and batch procedures. I tried to loop through the sequences and create 30% of the sequences for each batch. Then I replaced the final score (the final value) of each batch in the D column at the end of the 30% (for example, if I had 60 sequences in one batch, I sliced out the first 20 sequences and placed the final value of column D (in row 60) at row 20 in column D). After the data preprocessing, I used cross-validation to train the deep learning model.
On the other hand, in the second process, I used the window operator. I looped through the values of the dataset and created a 30% time step window for each batch. Then I placed the final value of each batch as a label for the 30% window. Then I used cross-validation to train the deep learning model.
I attached both processes, as well as a sample of my dataset. Therefore, I seek your advice regarding my concerns, which are:
- Is there any overall advice regarding these two processes?
- Is it allowed to use the windowing approach for preprocessing sequential data, even though it is often used for date and time series data?
- During model training using both processes, I faced an issue with the cross-validation performance results. I got a low squared correlation value; however, the relative error and the RMSE values were good. Is there any justification for this issue?
- My issue with the two processes is that I usually get a low squared correlation value when I train the RNN model or LSTM. Is there any advice that could help me enhance the performance results in terms of RMSE, RE, and squared correlation?
- Is there any advice that can help me handle the issue of getting good performance results using unseen data but bad results in the cross-validation performance results?
0