score metrics optimization other than accuracy
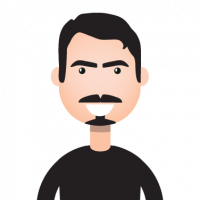

Hi,
1. Is it possible in RapidMiner to optimize score metrics other than accuracy (for a classification problem), that
is find the best parameters combinaison which maximize one of the score metrics of a Performance operator
(for example Recall or Precision etc.).
For the moment, I'm using an "handwork method" : I 'm using the Optimize Parameters (Grid) results, and then
I'm classing in descending order the column associated to the score metric I want to maximize and then I have access
to the associated parameters for the best performance.
2. I report that the results from Log operator (connected to perf output) are not the same that the results from
Optimize Parameters (Grid) . Is it normal ? (It seems that the results from Log operator are results from one of the iterations of cross validation operator.)
Here the process (dataset in attached zip file) :
<?xml version="1.0" encoding="UTF-8"?><process version="8.0.001">
<context>
<input/>
<output/>
<macros/>
</context>
<operator activated="true" class="process" compatibility="6.0.002" expanded="true" name="Process">
<process expanded="true">
<operator activated="true" breakpoints="after" class="read_excel" compatibility="8.0.001" expanded="true" height="68" name="Read Excel" width="90" x="179" y="34">
<parameter key="excel_file" value="C:\Users\Lionel\Documents\Formations_DataScience\Rapidminer\RapidMiner_Use_Cases\test_DT_unbalanced_data.xlsx"/>
<parameter key="imported_cell_range" value="A1:V79"/>
<parameter key="first_row_as_names" value="false"/>
<list key="annotations">
<parameter key="0" value="Name"/>
</list>
<list key="data_set_meta_data_information">
<parameter key="0" value="sex.true.integer.attribute"/>
<parameter key="1" value="family_status.true.integer.attribute"/>
<parameter key="2" value="pays_fee.true.integer.attribute"/>
<parameter key="3" value="customer_id.true.integer.attribute"/>
<parameter key="4" value="occupation.true.integer.attribute"/>
<parameter key="5" value="income.true.integer.attribute"/>
<parameter key="6" value="average_account_duration.true.real.attribute"/>
<parameter key="7" value="customer_for_years.true.real.attribute"/>
<parameter key="8" value="cash_withdrawals_sum.true.numeric.attribute"/>
<parameter key="9" value="income_sum.true.numeric.attribute"/>
<parameter key="10" value="insurance_sum.true.numeric.attribute"/>
<parameter key="11" value="creditcard_sum.true.numeric.attribute"/>
<parameter key="12" value="cash_withdrawals_avg.true.numeric.attribute"/>
<parameter key="13" value="income_avg.true.numeric.attribute"/>
<parameter key="14" value="insurance_avg.true.numeric.attribute"/>
<parameter key="15" value="creditcard_avg.true.numeric.attribute"/>
<parameter key="16" value="no_of_ch01_accounts.true.integer.attribute"/>
<parameter key="17" value="no_of_ch02_accounts.true.integer.attribute"/>
<parameter key="18" value="no_of_ch03_accounts.true.integer.attribute"/>
<parameter key="19" value="overdraft_total.true.integer.attribute"/>
<parameter key="20" value="no_of_accounts.true.integer.attribute"/>
<parameter key="21" value="is_buyer.true.polynominal.attribute"/>
</list>
</operator>
<operator activated="true" class="set_role" compatibility="8.0.001" expanded="true" height="82" name="Set Role" width="90" x="380" y="34">
<parameter key="attribute_name" value="is_buyer"/>
<parameter key="target_role" value="label"/>
<list key="set_additional_roles"/>
</operator>
<operator activated="true" class="concurrency:optimize_parameters_grid" compatibility="8.0.001" expanded="true" height="124" name="Optimize Parameters (Grid)" width="90" x="715" y="34">
<list key="parameters">
<parameter key="Set Macro.value" value="1,2,3,4,5,6,7,8,9,10"/>
<parameter key="Set Macro (2).value" value="1,2,3,4,5,6,7,8,9,10"/>
</list>
<parameter key="log_all_criteria" value="true"/>
<process expanded="true">
<operator activated="true" class="x_validation" compatibility="8.0.001" expanded="true" height="124" name="Validation" width="90" x="380" y="34">
<parameter key="number_of_validations" value="5"/>
<parameter key="sampling_type" value="shuffled sampling"/>
<process expanded="true">
<operator activated="true" class="set_macro" compatibility="8.0.001" expanded="true" height="82" name="Set Macro" width="90" x="45" y="34">
<parameter key="macro" value="weight_1"/>
<parameter key="value" value="10"/>
</operator>
<operator activated="true" class="set_macro" compatibility="8.0.001" expanded="true" height="82" name="Set Macro (2)" width="90" x="179" y="34">
<parameter key="macro" value="weight_0"/>
<parameter key="value" value="1"/>
</operator>
<operator activated="true" class="metacost" compatibility="8.0.001" expanded="true" height="82" name="MetaCost" width="90" x="380" y="34">
<parameter key="cost_matrix" value="[0.0 1.0;1.0 0.0]"/>
<process expanded="true">
<operator activated="true" class="concurrency:parallel_decision_tree" compatibility="8.0.001" expanded="true" height="103" name="Decision Tree" width="90" x="313" y="34"/>
<connect from_port="training set" to_op="Decision Tree" to_port="training set"/>
<connect from_op="Decision Tree" from_port="model" to_port="model"/>
<portSpacing port="source_training set" spacing="0"/>
<portSpacing port="sink_model" spacing="0"/>
</process>
</operator>
<connect from_port="training" to_op="Set Macro" to_port="through 1"/>
<connect from_op="Set Macro" from_port="through 1" to_op="Set Macro (2)" to_port="through 1"/>
<connect from_op="Set Macro (2)" from_port="through 1" to_op="MetaCost" to_port="training set"/>
<connect from_op="MetaCost" from_port="model" to_port="model"/>
<portSpacing port="source_training" spacing="0"/>
<portSpacing port="sink_model" spacing="0"/>
<portSpacing port="sink_through 1" spacing="0"/>
</process>
<process expanded="true">
<operator activated="true" class="apply_model" compatibility="7.1.001" expanded="true" height="82" name="Apply Model to Testset" width="90" x="45" y="30">
<list key="application_parameters"/>
</operator>
<operator activated="true" class="performance_binominal_classification" compatibility="8.0.001" expanded="true" height="82" name="Performance" width="90" x="179" y="34">
<parameter key="precision" value="true"/>
<parameter key="recall" value="true"/>
<parameter key="f_measure" value="true"/>
<parameter key="false_positive" value="true"/>
<parameter key="false_negative" value="true"/>
<parameter key="true_positive" value="true"/>
<parameter key="true_negative" value="true"/>
</operator>
<connect from_port="model" to_op="Apply Model to Testset" to_port="model"/>
<connect from_port="test set" to_op="Apply Model to Testset" to_port="unlabelled data"/>
<connect from_op="Apply Model to Testset" from_port="labelled data" to_op="Performance" to_port="labelled data"/>
<connect from_op="Performance" from_port="performance" to_port="averagable 1"/>
<portSpacing port="source_model" spacing="0"/>
<portSpacing port="source_test set" spacing="0"/>
<portSpacing port="source_through 1" spacing="0"/>
<portSpacing port="sink_averagable 1" spacing="0"/>
<portSpacing port="sink_averagable 2" spacing="0"/>
</process>
</operator>
<operator activated="true" class="log" compatibility="8.0.001" expanded="true" height="82" name="Log" width="90" x="581" y="85">
<list key="log">
<parameter key="DT_accuracy" value="operator.Performance.value.accuracy"/>
<parameter key="DT_recall" value="operator.Performance.value.recall"/>
<parameter key="DT_precision" value="operator.Performance.value.precision"/>
<parameter key="DT_TP" value="operator.Performance.value.true_positive"/>
<parameter key="DT_TN" value="operator.Performance.value.true_negative"/>
<parameter key="DT_FP" value="operator.Performance.value.false_positive"/>
<parameter key="DT_FN" value="operator.Performance.value.false_negative"/>
<parameter key="DT_F" value="operator.Performance.value.f_measure"/>
</list>
</operator>
<connect from_port="input 1" to_op="Validation" to_port="training"/>
<connect from_op="Validation" from_port="model" to_port="model"/>
<connect from_op="Validation" from_port="averagable 1" to_op="Log" to_port="through 1"/>
<connect from_op="Log" from_port="through 1" to_port="performance"/>
<portSpacing port="source_input 1" spacing="0"/>
<portSpacing port="source_input 2" spacing="0"/>
<portSpacing port="sink_performance" spacing="0"/>
<portSpacing port="sink_model" spacing="0"/>
<portSpacing port="sink_output 1" spacing="0"/>
</process>
</operator>
<operator activated="true" class="read_excel" compatibility="8.0.001" expanded="true" height="68" name="Read Excel (2)" width="90" x="179" y="595">
<parameter key="excel_file" value="C:\Users\Lionel\Documents\Formations_DataScience\Rapidminer\RapidMiner_Use_Cases\test_DT_unbalanced_data.xlsx"/>
<parameter key="imported_cell_range" value="A1:V79"/>
<parameter key="first_row_as_names" value="false"/>
<list key="annotations">
<parameter key="0" value="Name"/>
</list>
<list key="data_set_meta_data_information">
<parameter key="0" value="sex.true.integer.attribute"/>
<parameter key="1" value="family_status.true.integer.attribute"/>
<parameter key="2" value="pays_fee.true.integer.attribute"/>
<parameter key="3" value="customer_id.true.integer.attribute"/>
<parameter key="4" value="occupation.true.integer.attribute"/>
<parameter key="5" value="income.true.integer.attribute"/>
<parameter key="6" value="average_account_duration.true.real.attribute"/>
<parameter key="7" value="customer_for_years.true.real.attribute"/>
<parameter key="8" value="cash_withdrawals_sum.true.numeric.attribute"/>
<parameter key="9" value="income_sum.true.numeric.attribute"/>
<parameter key="10" value="insurance_sum.true.numeric.attribute"/>
<parameter key="11" value="creditcard_sum.true.numeric.attribute"/>
<parameter key="12" value="cash_withdrawals_avg.true.numeric.attribute"/>
<parameter key="13" value="income_avg.true.numeric.attribute"/>
<parameter key="14" value="insurance_avg.true.numeric.attribute"/>
<parameter key="15" value="creditcard_avg.true.numeric.attribute"/>
<parameter key="16" value="no_of_ch01_accounts.true.integer.attribute"/>
<parameter key="17" value="no_of_ch02_accounts.true.integer.attribute"/>
<parameter key="18" value="no_of_ch03_accounts.true.integer.attribute"/>
<parameter key="19" value="overdraft_total.true.integer.attribute"/>
<parameter key="20" value="no_of_accounts.true.integer.attribute"/>
<parameter key="21" value="is_buyer.true.polynominal.attribute"/>
</list>
</operator>
<operator activated="true" class="python_scripting:execute_python" compatibility="7.4.000" expanded="true" height="82" name="Execute Python" width="90" x="380" y="595">
<parameter key="script" value="import pandas as pd from sklearn.preprocessing import LabelEncoder # rm_main is a mandatory function, # the number of arguments has to be the number of input ports (can be none) def rm_main(data): #X = data.iloc[:,0:23] le = LabelEncoder() data.iloc[:,21] = le.fit_transform(data.iloc[:,21]) # connect 2 output ports to see the results return data"/>
</operator>
<operator activated="true" class="python_scripting:execute_python" compatibility="7.4.000" expanded="true" height="103" name="Execute Python (2)" width="90" x="514" y="595">
<parameter key="script" value="import pandas as pd from sklearn.tree import DecisionTreeClassifier from sklearn.model_selection import cross_val_score # rm_main is a mandatory function, # the number of arguments has to be the number of input ports (can be none) def rm_main(data): X = data.iloc[:,0:21] y = data.iloc[:,21] #DT = DecisionTreeClassifier(class_weight = 'balanced') DT = DecisionTreeClassifier(class_weight = {0:1,1:10}) #DT = DecisionTreeClassifier() DT.fit(X,y) acc = (100*cross_val_score(DT,X,y, scoring = 'recall_micro',cv = 10)).mean() accuracy = pd.DataFrame(data = [acc],columns = ['recall micro']) # connect 2 output ports to see the results return data,accuracy"/>
</operator>
<connect from_op="Read Excel" from_port="output" to_op="Set Role" to_port="example set input"/>
<connect from_op="Set Role" from_port="example set output" to_op="Optimize Parameters (Grid)" to_port="input 1"/>
<connect from_op="Optimize Parameters (Grid)" from_port="performance" to_port="result 5"/>
<connect from_op="Optimize Parameters (Grid)" from_port="model" to_port="result 1"/>
<connect from_op="Optimize Parameters (Grid)" from_port="parameter set" to_port="result 2"/>
<connect from_op="Read Excel (2)" from_port="output" to_op="Execute Python" to_port="input 1"/>
<connect from_op="Execute Python" from_port="output 1" to_op="Execute Python (2)" to_port="input 1"/>
<connect from_op="Execute Python (2)" from_port="output 1" to_port="result 3"/>
<connect from_op="Execute Python (2)" from_port="output 2" to_port="result 4"/>
<portSpacing port="source_input 1" spacing="0"/>
<portSpacing port="sink_result 1" spacing="0"/>
<portSpacing port="sink_result 2" spacing="0"/>
<portSpacing port="sink_result 3" spacing="0"/>
<portSpacing port="sink_result 4" spacing="0"/>
<portSpacing port="sink_result 5" spacing="0"/>
<portSpacing port="sink_result 6" spacing="0"/>
</process>
</operator>
</process>
Thanks you for your responses.
Regards,
Lionel
Best Answer
-
Thomas_Ott RapidMiner Certified Analyst, RapidMiner Certified Expert, Member Posts: 1,761
Unicorn
Yes, you can optimize on kappa, F1, and all the other metrics available in whatever performance operator you use. Just make sure to toggle on the one you want and it'll optimize on that measure.
2
Answers
Hi @Thomas_Ott
Thanks you for your fast response. I did not understand your response right away : I had to choose my score metric to optimize
in the main criterion parameter of the Performance operator.
Regards,
Lionel
Yes, that;'s what I meant. Not enough coffee yet.